The mother’s arm is moving upwards: how are we able to see in her gesture the tender sweetness leading her to caress the cheek of her sleeping child or the inner violence preparing to hit the soldier’s cheek? How can we foresee in the heavy murderer’s hand movement a slight faltering revealing his fragile uncertainty in finishing his task, how can we measure the hesitation of the woman’s arms closing to protect her crying, in resonant dialogue with the bodies moving around her? Understanding, measuring and predicting the qualities of movement imply a dynamic cognitive relation with a complex non-linearly stratified temporal dimension. Movements are hierarchically nested: a gesture sequence has a hierarchical layered structure: from high level layers down to more and more local components (as a language: from syntax to phonemes) where every layer influences and is influenced by every other (bottom-up/top-down). Every layer is characterized by a different temporal dimension: a proper rhythm from macro to micro temporal scales of action. This organization does not only apply to action execution, but also to action observation and is at the basis of the unique human ability to understand and predict conspecific gestural qualities. Human skill in understanding and predicting gestural qualities, and attempting to influence one another’s actions, depends on the capacity to create intercrossing relations between these different temporal and spatial layers through feedforward/feedback connections and bidirectional causalities, with the body as a time keeper, coordinating different internal, mental and physiological clocks. In 1973, Johansson showed that the human visual system can perceive the movement of a human body from a limited number of moving points. This landmark study grounded the scientific bases of current motion capture technologies. Recent studies proved that the information contained in such a limited number of moving points does not concern only the activity performed, but can also provide hints about more complex cognitive and affective phenomena: for example, Pollick (2001) showed that participants can infer emotional categories from point-light representations of everyday actions). Studies using naturalistic images and videos have established how fluent we are in body language (de Gelder, 2016). Very few studies consider the temporal dynamics of the stimulus, and how affective qualities may be perceived faster than other qualities (Meeren et al 2016), be interlinked and change over time. In other words, time is a crucial variable for these processes. Such time intervals are the time intervals of human perception and prediction, i.e., this is a human time, which integrates time at the neural level up to time at the level of narrative structures and content organization. Current technologies either do not deal with such a human time or they do in a quite empirical way: motion capture technologies are most often limited to computation of kinematic measures whose time frame is usually too short for an effective perception and prediction of complex phenomena. While a lot of effort is being spent improving such technologies in the direction of more accurate and more portable systems (e.g., wearable and wireless), such developments are incremental with respect to a conceptual and technological paradigm that remains unchanged. Furthermore, most systems for gesture recognition or for analysis of emotional content from movement data streams adopt time processing windows whose duration is fixed and is usually empirically determined. EnTimeMent proposes a radical change of paradigm and technology in human movement analysis, where the time frame for analysis is grounded on novel neuroscientific, biomechanical, psychological, and computational evidence, and dynamically adapted to the human time governing the phenomena under investigation. This is obtained through innovative scientifically-grounded and time-adaptive technologies that can operate at multiple time scales in a multi-layered approach, transforming the current generation of motion capture and movement analysis systems and endowing them with a completely novel functionality to achieve a novel generation of time-aware multisensory motion perception and prediction systems. The goal of EnTimeMent is to develop the scientific knowledge and the enabling technologies for such a transformation. EnTimeMent will enable novel forms of human-machine interaction (human-machine affective synchronization) and human-to-human entrainment experiences, primarily concerned with the non-verbal, embodied and immersive, active and affective dimensions of qualitative gesture. For instance, novel machine learning techniques inspired by deep networks (Bengio, 2015) might afford the detection of patterns in motion to represent motion at gradually increasing levels of abstraction using Empirical Mode Decomposition (Huang et al., 1998) to extract local characteristic and time scale of the data automatically. This will effectively reduce the originally high-dimensional and redundant raw sensor observations to something that is more manageable for inference of, for example, emotions. The experimental platform will integrate signals from sensors capturing movement in relation to respiration, heart rate, muscle activity, sound, and brain activity across multiple time scales. By these means, the main technological breakthrough of EnTimeMent will be promoting novel perspectives on understanding, measuring and predicting the qualities of movement (at individual and group level) in motion capture, multisensory interfaces, wearables, affective and IoT technologies.
|
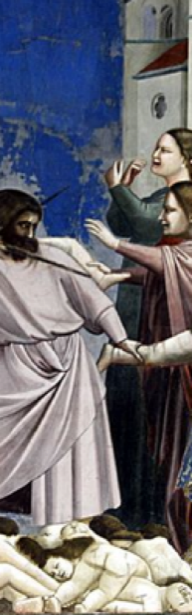
|